AI Garbage Sorting Using Object Detection CNN
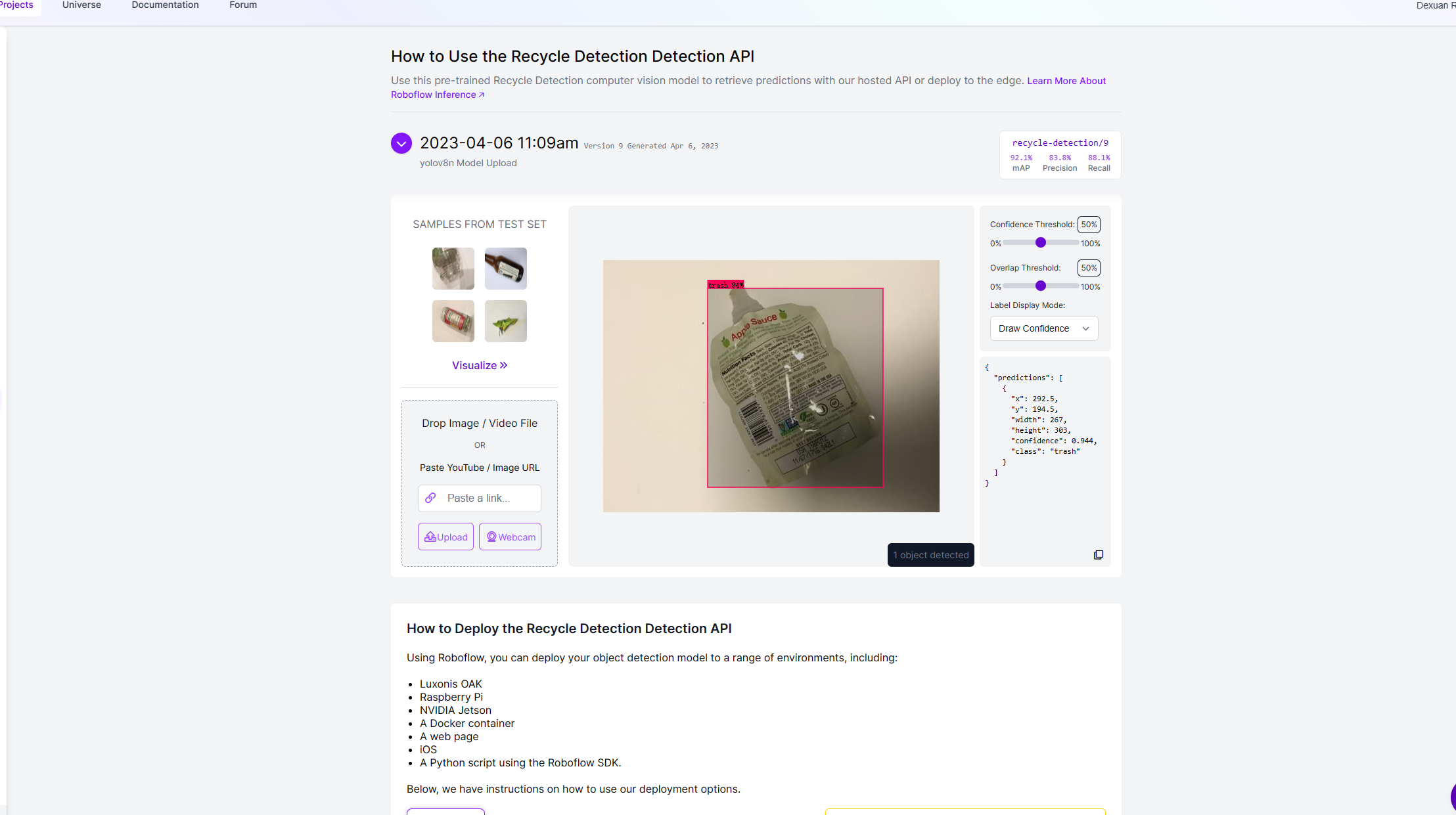
Title: CNN Thunderdome Showdown: Benchmarking YOLOv7, VGG-16, and GoogleNet for Recyclables Image Classification Accuracy
Date: April 8, 2023
Authors: Tom Sun, Dexuan Ren, Deepta Adhikary
University: York University
Abstract
The study focuses on image classification for recyclable sorting and classification. Three models were tested: YOLOv7, VGG-16, and GoogleNet. GoogleNet was found to be the most effective with an accuracy rate of 94% and faster training speed. The findings can be applied to reduce costs in recycling plants and create garbage collecting mini robots.
Introduction
The project explores the challenges of image classification and how transfer learning using pre-trained models like CNNs can overcome these challenges. The study focuses on recyclables and assumes that recycling plants have techniques to isolate each piece of recyclable and capture images.
Methodology
- Models Used: YOLOv7, VGG-16, and GoogleNet.
- Dataset: Kaggle dataset for recyclables.
- Design & Training Pipeline: Pre-trained VGG-16 and GoogleNet models implemented in PyTorch. YOLOv7 used for object detection. Images processed using RoboFlow platform.
- Model Training Changes for YOLOv7: Background removal and manual relabeling.
Results
- Baseline Accuracy: 70% (average from Kaggle models), Human accuracy at 99%.
- Scores:
- YOLOv7: 70%
- VGG-16: 88%
- GoogleNet: 94%
- Observations: Different models have strengths and weaknesses depending on the specific materials or objects being classified.
Discussion
- Strengths and Limitations: YOLOv7 required significant overhead, while VGG-16 and GoogleNet were easier to train. GoogleNet was concluded as the better model for this task.
- Future Directions: Further testing on various datasets and exploring other areas like facial or shape recognition.
- Peer Evaluation: Feedback received and changes made to improve the report.
Updated YOLO V8 and latest model
The project has been updated to use the latest YOLO V8, which enhances the performance and efficiency of the models. You can find more details about YOLO V8 model here.
Conclusion
The study provides a foundation for automated garbage sorting, potentially reducing costs and human effort in waste management. GoogleNet was identified as the most suitable model for this task, with potential applications in recycling industries and the creation of waste-collecting robots.
comment:
Get In Touch
Feel free to reach out to me with any questions, feedback, or collaboration opportunities. I would love to hear from you!